Unveiling the Power of Machine Learning: Transforming Data into Intelligence
Share this Post to earn Money ( Upto ₹100 per 1000 Views )
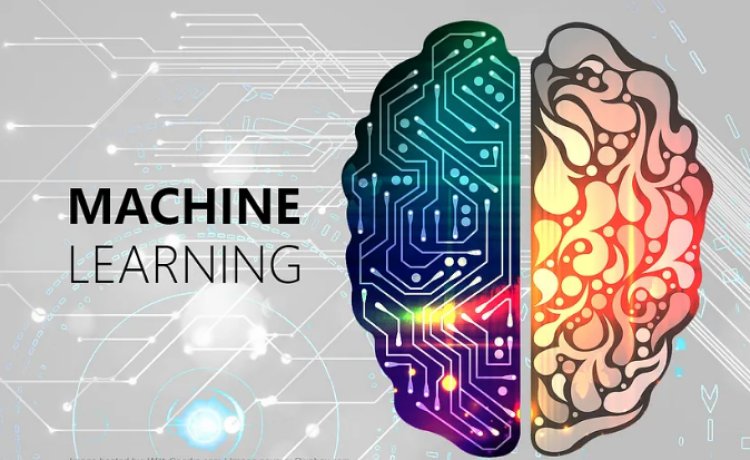
Introduction
Machine Learning (ML) has emerged as a groundbreaking technology that is reshaping the way we interact with data and make decisions. Rooted in artificial intelligence (AI), Machine Learning is a dynamic field that enables computers to learn from data and improve their performance over time without explicit programming. This transformative technology is permeating various industries, from healthcare and finance to marketing and beyond, offering new possibilities and solutions to complex problems.
Understanding Machine Learning
At its core, machine learning is a data-driven approach that allows computers to recognize patterns, make predictions, and optimize decisions. Unlike traditional programming, where developers provide explicit instructions, ML algorithms learn from data, adapting and evolving their behavior based on the information they process. This ability to learn from experience empowers machines to handle diverse and intricate tasks, ranging from image recognition and natural language processing to recommendation systems and autonomous vehicles.
Types of Machine Learning
Machine learning can be broadly categorized into three types:
- Supervised Learning: In supervised learning, the algorithm is trained on a labeled dataset, where the input data and corresponding output are provided. The model learns to map the input data to the correct output, making predictions on new, unseen data.
- Unsupervised Learning: Unsupervised learning deals with unlabeled data, where the algorithm must identify patterns and relationships without explicit guidance. Clustering and dimensionality reduction are common tasks in unsupervised learning.
- Reinforcement Learning: In reinforcement learning, an agent learns to make decisions by interacting with an environment. The agent receives feedback in the form of rewards or penalties, allowing it to adjust its behavior and improve over time.
Applications of Machine Learning
The applications of machine learning are vast and continue to expand across industries. Some notable examples include:
- Healthcare: ML is transforming healthcare with applications like disease diagnosis, personalized treatment plans, and drug discovery. Algorithms can analyze medical images, predict patient outcomes, and assist in early detection.
- Finance: In the financial sector, machine learning is used for fraud detection, credit scoring, algorithmic trading, and risk management. ML models analyze vast amounts of financial data to make informed decisions.
- Marketing and E-Commerce: ML powers recommendation engines, customer segmentation, and targeted advertising in the marketing and e-commerce sectors. This enhances user experience and improves conversion rates.
- Autonomous Vehicles: The development of self-driving cars relies heavily on machine learning algorithms for tasks such as object recognition, path planning, and real-time decision-making.
Challenges and Considerations
While machine learning holds immense potential, it comes with its set of challenges. Some of the key considerations include:
- Data Quality and Bias: ML models heavily depend on the quality of the training data. Biases present in the data can lead to biased predictions, reinforcing existing inequalities.
- Interpretability: Understanding and interpreting the decisions made by complex ML models can be challenging. This lack of interpretability raises concerns, especially in critical applications like healthcare and finance.
- Scalability: As datasets and computational requirements grow, scalability becomes a crucial factor. Developing scalable ML solutions that can handle large volumes of data is an ongoing challenge.
Conclusion
Machine learning is a transformative force that is revolutionizing the way we leverage data for decision-making. As the field continues to advance, addressing challenges such as bias, interpretability, and scalability will be essential for realizing its full potential. With ongoing research and innovation, machine learning is set to drive further advancements across industries, unlocking new possibilities and reshaping the future of technology.