The Importance of Statistical Significance
Understanding statistical significance not only deepens your analytical skills but is also invaluable in assignments. Economics assignment help provide support for students who wish to delve into this topic.
Share this Post to earn Money ( Upto ₹100 per 1000 Views )
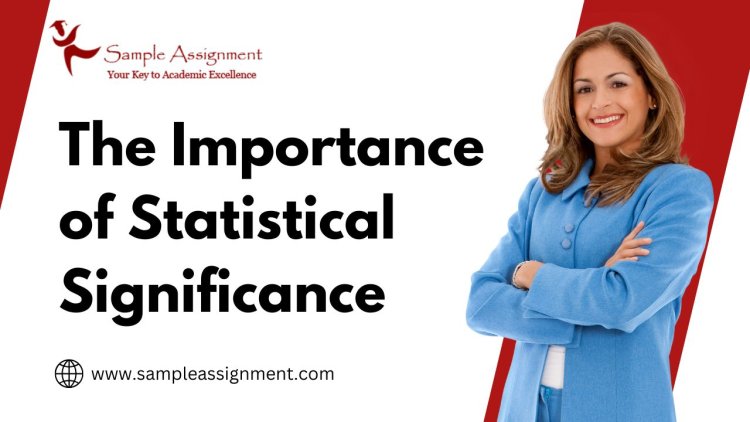
In academic research and various industries, statistical significance is a fundamental concept that helps ensure that results and conclusions are meaningful and not merely due to random chance. This is especially relevant for students in fields like economics and statistics, where interpreting data correctly is crucial. This post explores what statistical significance is, why it matters, and how to determine it in research settings.
Understanding statistical significance not only deepens your analytical skills but is also invaluable in assignments. Resources like economics assignment help provide support for students who wish to delve into this topic or seek clarity in their coursework.
What is statistical significance?
Statistical significance is a quantitative measure used by researchers that helps them test the hypothesis or correlation arrived at, whether it resulted from a particular factor or by chance. In simple terms, the likelihood that any observed effect in the study isn’t due to chance is high if the result is statistically significant. The likelihood of getting the observed result by chance alone is calculated by the p-value, while a small p-value suggests a statistically significant result.
For instance, in a research endeavour whose purpose is to determine whether a new method of teaching enhances students’ performance. The test of statistical significance explains whether any variation in performance is really attributable to the enhancement or is just an event of chance.
For students on assignments or research papers, there is no better way of boosting their work credibility than to achieve the set statistical significance. Especially in the areas like economics, statistic and social sciences, it is helpful to understand the significance testing.
When students are stuck on how to compute or even interpret such statistical significances, getting a statistics assignment help Australia to guide them can be very helpful as they make learning such statistics a lot easier.
The Role of Statistical Significance in Economics
In fact, in the broader perspective of economics, where decisions have consequences in policy formulation or management of large populations. Statistical significance is a tool used to assist the analyst/researcher in making the correct decisions. They also allow the identification of the real economic drivers from noise, thus enhancing the credibility of forecasts and models. Here’s how statistical significance impacts key areas in economics:
Economic Forecasting
When it comes to future trends, such as the unemployment rate or GDP growth, economists will use past data and different methods. Such data must show statistical significance in its results, and this assists economists in making forecasts of future conditions. In the context of learners working on assignments related to forecasting, the economics assignment help is really going to help them understand how to statistically approach these trends.
Policy Analysis
Generally, statistical significance tests are employed to compare the efficiency of government policies in policy studies. For instance, while investigating the relationship between tax cuts and spending, a significant result suggests a probable influence helping the policymakers.
Financial Markets
In finance, it has the advantage of identifying genuine market trends with regard to random fluctuations of the read full. A notable discovery may indicate that a particular alteration, such as a change in interest rates. It really causes stock prices to change.
Interpreting Statistical Significance: Avoiding Misinterpretations
Although higher statistical significance is always a good thing, there are indeed catches with this measure. Here are some key considerations when interpreting significance:
Statistical vs. Practical Significance:
Therefore, a statistically significant result may not always be practically significant. For instance, when comparing two groups, a truly tiny difference may be statistically significant but not practically significant—a difference present in numbers but not in meaningful substance.
Sample Size Dependence:
ERROR means of large samples can be statistically significant even in the existence of minor effects. Conversely, ERROR means of small samples could not be statistically significant even if an effect exists.
Significance Does Not Imply Causation:
The results obtained can be statistically significant but they are not enough to draw cause-and-effect relations between the factors examined.
Why Statistical Significance Matters
Understanding statistical significance is crucial for several reasons:
Informed Decision-Making:
Statistical significance is useful in research, as well as in the social sciences and economics, to make necessary adjustments and remove the “noise” interference that makes the results obtainable.
Confidence in Results:
Statistical significance ensures that the researcher can pin the findings on something rather than the outcome being a mere accident.
Reproducibility of Research:
Learned results are normally more manyfold reproducible than significant, which signifies that the results can be tested by other researchers.
Common Pitfalls in Using Statistical Significance
The pitfalls are as follows:
Over-Reliance on P-Values
P-values are important but a number of problems can arise when they are used excessively. One may answer could be statistically significant but practically insignificant.
Ignoring Effect Size
Cohen’s d is an index of the size of an effect, not just as important as the statistical significance of an effect. For instance, a change in the consumer spending fraction can be very small yet big enough statistically to warrant policy adjustment at that fraction.
Data Snooping
Performing multiple hypotheses tests on a single data set means that there is a high likelihood of obtaining a statistically significant result by mere coincidence. This is called data snooping and can result in coming to wrong conclusions.
Sample Size Bias
Population sizes overwhelmingly impact statistical significance. Large groups, when tested, may present a p value that depicts a significant difference, however trivial, while smaller groups may be harmless despite depicting relevance differences.
Conclusion
If statistical significance is a term, it is more than that. It is the pillar that stands at the core of the economy and the teaching of statistics. The lessons, therefore, help the student or the researcher extract meaningful information from the test results that is statistically significant.
No matter what it is that you are researching—economics, statistics, or any other type of data-based paper—understanding statistical significance can only work in your favour and make your arguments more legitimate. If statistical analysis is hard for you to go through, there is always assignment help online available to give a specialized approach.
By gaining knowledge regarding statistical significance, one not only gains higher grades but also obtains valuable experience of a career in today's world, as existing hi-tech realms of professional activity are based and built on data analysis and decision-making them. Data analysis is a critically important skill for any field, from economics and marketing to social sciences in general. Therefore, welcome the process of becoming a master of statistical significance and use that knowledge in every data decision you make!