How to Leverage Data for Quality Assurance Best Practices
Share this Post to earn Money ( Upto ₹100 per 1000 Views )
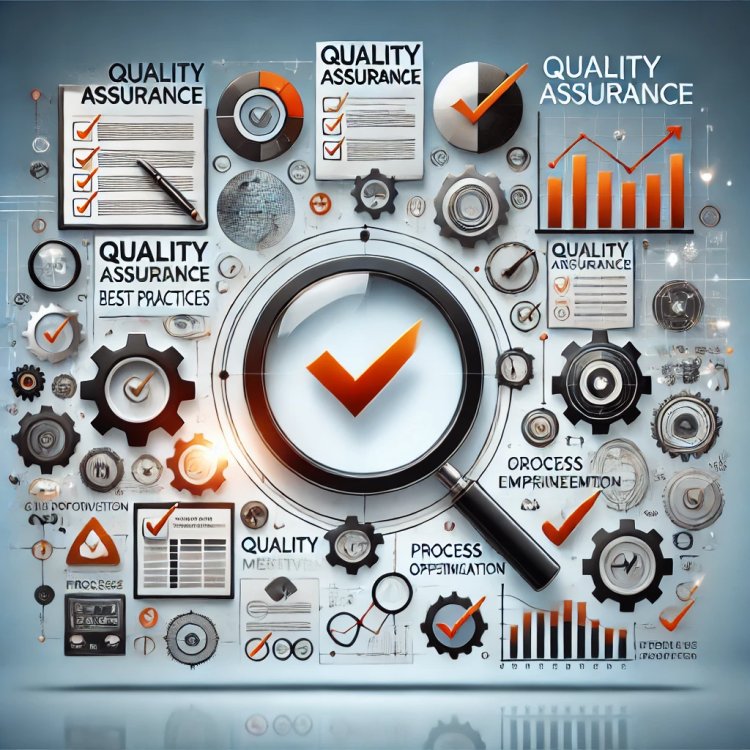
In today’s competitive and highly regulated industries, leveraging data effectively has become integral to implementing Quality Assurance Best Practices. From enhancing decision-making to ensuring compliance and improving operational efficiency, data-driven approaches provide the foundation for achieving excellence in quality management. Organizations in life sciences, manufacturing, and other regulated sectors can optimize their quality management systems by adopting qa standards and best practices that focus on data utilization. This blog explores how organizations can use data to implement quality assurance methodologies that lead to superior outcomes.
1. The Role of Data in Quality Assurance Best Practices
1.1 Understanding the Importance of Data in QA Practices
Data plays a critical role in modern quality assurance methodologies by providing actionable insights that support continuous improvement. Organizations can leverage data to identify trends, track performance, and predict potential issues before they arise. Quality assurance best practices rely on accurate data collection and analysis to ensure products meet regulatory standards and customer expectations.
1.2 How QA Standards and Best Practices Depend on Data
Data-driven qa standards and best practices ensure that every process in the quality management system is optimized for efficiency and effectiveness. By integrating data analytics tools into their quality assurance strategies, organizations can improve product quality, minimize errors, and achieve compliance with industry regulations.
2. Building a Data-Driven Quality Management System
2.1 Establishing Metrics for QA Best Practices
Implementing a data-driven quality management system begins with defining key performance indicators (KPIs) that align with organizational goals. These metrics help track progress toward meeting qa standards and best practices. Examples of useful metrics include defect rates, on-time delivery, and customer satisfaction scores.
2.2 Leveraging QMS Platforms for Data Integration
A robust QMS integrates data from various sources across the organization, providing a centralized platform for analysis and reporting. By consolidating data, organizations can ensure consistency and accuracy, which are essential for maintaining quality assurance best practices.
3. Collecting and Analyzing Quality Data
3.1 Methods for Data Collection in QA Practices
Effective Quality Assurance methodologies require accurate and comprehensive data collection methods. Organizations can collect data from production lines, customer feedback, audits, and compliance reports. Advanced QMS tools and IoT-enabled devices facilitate real-time data collection, ensuring timely insights.
3.2 Using Analytics to Drive QA Best Practices
Data analytics plays a pivotal role in identifying patterns and uncovering areas for improvement. By analyzing historical and real-time data, organizations can implement targeted interventions to address quality issues, optimize workflows, and ensure compliance with qa standards and best practices.
4. Enhancing Decision-Making with Data-Driven QA Standards
4.1 Real-Time Reporting for Quality Assurance Methodologies
Real-time reporting enables organizations to make informed decisions based on current data. Quality assurance best practices emphasize the use of dashboards and automated reports to provide managers with actionable insights that support continuous improvement.
4.2 Predictive Analytics for Preventive QA Practices
Predictive analytics allows organizations to anticipate potential quality issues before they occur. By leveraging data trends and advanced algorithms, organizations can implement preventive measures that align with qa methodologies, reducing defects and ensuring compliance.
5. Driving Continuous Improvement Through QA Standards and Best Practices
5.1 Implementing Feedback Loops in Quality Management
Continuous improvement is a cornerstone of quality assurance best practices. Feedback loops supported by data-driven insights allow organizations to refine processes, address gaps, and enhance product quality. A QMS enables organizations to track and analyze feedback effectively.
5.2 Aligning Continuous Improvement with QA Practices
By integrating continuous improvement initiatives into their quality assurance methodologies, organizations can adapt to changing market demands and regulatory requirements. Data-driven decision-making ensures that qa standards and best practices remain relevant and effective.
6. Ensuring Compliance with Data-Driven QA Methodologies
6.1 Using Data to Meet Regulatory Standards
Regulatory compliance is a critical component of quality assurance best practices. Organizations can use data to demonstrate compliance with industry standards, including FDA regulations, ISO certifications, and GMP requirements. QMS platforms help automate compliance tracking and ensure that records are audit-ready.
6.2 Automating Compliance Efforts with QMS Tools
QMS tools equipped with data analytics capabilities streamline compliance efforts by automating documentation, monitoring regulatory changes, and generating reports. This data-driven approach ensures organizations stay ahead of compliance challenges while maintaining qa standards.
7. Training and Empowering Teams for Data-Driven QA Practices
7.1 Building Data Competency in QA Teams
Successful implementation of quality assurance best practices requires teams to be proficient in data collection, analysis, and interpretation. Training programs that focus on data literacy and QMS tools empower employees to contribute to quality initiatives effectively.
7.2 Using Data to Enhance Employee Performance
Organizations can use data to monitor employee performance and identify areas for skill development. By aligning training programs with data insights, companies can ensure their teams are equipped to uphold qa standards and implement best practices consistently.
8. Leveraging Technology for Quality Assurance Methodologies
8.1 Integrating AI and Machine Learning into QA Practices
Artificial intelligence (AI) and machine learning (ML) technologies enhance quality assurance methodologies by automating data analysis and identifying patterns. These tools help organizations optimize processes, predict defects, and ensure compliance with qa best practices.
8.2 Using Cloud-Based QMS Solutions for Scalability
Cloud-based QMS Solutions provide organizations with the flexibility to scale their quality assurance efforts across multiple locations. These QMS solutions ensure that data is accessible in real-time, facilitating collaboration and consistency in implementing quality assurance best practices.
Conclusion
Leveraging data effectively is fundamental to implementing quality assurance best practices that drive operational excellence and ensure compliance. By adopting data-driven approaches, organizations can enhance decision-making, optimize processes, and achieve continuous improvement.
ComplianceQuest Management Software provides a robust solution for overseeing quality assurance methodologies, enhanced by cutting-edge data analytics capabilities. Its cloud-based QMS platform enables organizations to collect, analyze, and act on quality data in real-time, ensuring adherence to qa standards and best practices. In 2024, investing in ComplianceQuest will empower businesses to achieve superior product quality, maintain compliance, and foster a culture of continuous improvement.